As shown, to show the whole feature set for a single example, we use x(1). We can also point to the first feature in third example in training set as X(3,1) or x1(3).
For simplicity of understanding, let's assume, there is only one feature in our dataset of 10 examples. Let's plot it on a graph.
def HypoThe(Theta,xi): if(len(Theta)==len(xi)): sum=0 for i in range(len(xi)): sum+=(Theta[i]*xi[i]) return sum else: return False
def RegCostFunc(Theta,X,Y): sum1=0 for i in range(len(X)): sum1+=((HypoThe(Theta, X[i])-Y[i])**2) J=sum1 return J/(2*len(X))
def GradTerm(X,Y,Theta,i): sum1=0 for j in range(len(X)): sum1+=((HypoThe(Theta,X[j])-Y[j])*X[j][i]) return sum1
def GradDesc(Theta,alpha,Xfeature,Ylabels): Theta_=[] for i in range(0,len(Theta)): Theta_.append(Theta(i)-(alpha* GradTerm(Xfeature,Ylabels,Theta,i)/len(Xfeature))) return Theta_
def LinearRegression(Xfeature,Ylabels,alpha,iterations): if len(Xfeature)!=len(Ylabels): print("Missing Data"); return False else: for i in range(len(Xfeature)): Xfeature[i].insert(0,1) Theta=[0]*len(Xfeature[0]) for i in range(iterations): print("\nIteration Number ",i) print(Theta) Theta=GradDesc(Theta, alpha, Xfeature, Ylabels) print(Theta) return Theta

Other Lessons for You
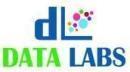
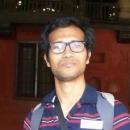
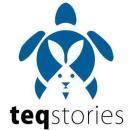
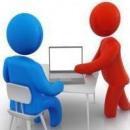
Find Machine Learning near you