Yeah, I know, it will take a moment to understand it. But it is the best definition you can encounter.
Let's understand it for a moment with some example.
Area (sq. mtrs) | Rooms | Price(USD) |
3890 | 3 | 573900 |
1100 | 3 | 249900 |
1458 | 3 | 464500 |

Other Lessons for You
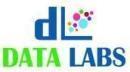
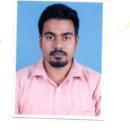
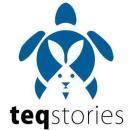
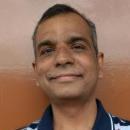
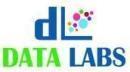
Find Machine Learning near you
Looking for Machine Learning ?
Learn from Best Tutors on UrbanPro.
Are you a Tutor or Training Institute?
Join UrbanPro Today to find students near you